Imagine asking an AI to draw a top hat on a table with a rabbit being pulled out of it—as in a classic magic trick—but instead, the hat ends up with the opening facing downward, firmly placed on the table. If that sounds perplexing, you’re not alone. Today, we’ll explore why image-generating AIs sometimes produce unexpected results, and we’ll also discuss the notorious difficulties these models face when drawing lifelike humans and maintaining symmetry in objects like cars.
How Do Image Generators Learn?
At the heart of these systems are deep neural networks trained on millions of images. Rather than memorizing individual pictures, these AIs learn common patterns, textures, and spatial relationships from vast datasets—a process that helps them build what’s known as a latent space. This internal map represents a complex blend of the elements found in images and serves as the foundation for generating new, original artwork. In essence, the AI has learned a “visual language” that it uses to craft its creations.
Training Data Biases: When Patterns Persuade
One major reason for unexpected outputs is the bias in the training data. If most images of a top hat in the dataset show it with a particular orientation, the AI may assume that orientation is “correct,” even when your instructions suggest otherwise. Similarly, if the dataset has many images of cars with a slight asymmetry or photos of humans with common facial positions, the AI will lean toward those learned patterns. The result is that the probabilistic nature of the AI pushes it to generate images based on what’s statistically common in its training material.
The Challenges of Ambiguous Prompts
Language is naturally open to interpretation. A description like “a top hat on a table with a rabbit being pulled out of it” leaves room for uncertainty. Without explicitly stating details—such as “with the hat’s opening facing upward so the rabbit can emerge”—the AI fills in the gaps based on its internalized patterns. This ambiguity can lead to errors in spatial arrangement, orientation, and even the proportions of the depicted elements.
Spatial Reasoning and Artistic Interpretation
Humans process spatial relationships intuitively. We instantly know that for a magic-act depiction, the hat should have its opening facing up. However, AI models struggle with this level of spatial reasoning. They work by independently synthesizing object details from a latent space, which sometimes results in inconsistencies in object placement and alignment. The randomness and probability that come with generating an image can occasionally lead to outcomes that defy our common sense—that’s why you might see a table-top hat drawn “backward.”
The Probabilistic Nature of Creativity
Every image an AI generates is the result of sampling from a probability distribution—a creative lottery of sorts. While this process often yields beautiful and novel creations, it also means that achieving exact reproducibility or precision can be elusive. Each run of a model, even with the same input prompt, might produce variations that reflect slight misinterpretations of spatial cues or object relationships.
The Complex Task of Drawing Humans
Humans are incredibly intricate subjects to depict. When drawing a human face or figure, even small errors—like misplacing an eye by a few pixels—can make the difference between a compelling portrait and an uncanny, almost off-putting image. This happens because our brains are wired to notice subtle asymmetries and irregularities in human anatomy. Moreover, human expressions, skin textures, and the interplay of light and shadow add layers of complexity that AI needs to learn and replicate. A slight fuzziness in the details or an extra finger on a hand is often immediately evident because our dataset or training methods might not have perfectly balanced all these elements. As a result, the state-of-the-art models sometimes struggle to bridge the gap between technical generation and the nuanced realism that human features demand.
Struggles With Symmetry in Image Generation
Just as humans are sensitive to imperfections in human features, we are also naturally tuned to recognize symmetry in objects we expect to be balanced. Cars, for instance, are designed to be symmetrical—each side mirroring the other—providing visual harmony and driving aesthetics. Yet AI models can falter in this area. Since image generators work by assembling details based on learned patterns, constructing a perfectly symmetrical object involves generating two halves in precise alignment. Even slight misalignments can result in noticeably asymmetrical designs, or parts of an object appearing off-balance. This difficulty is rooted in the fact that while the model excels in creating elements that are “good enough” on their own, integrating them into a unified, perfectly balanced whole poses a unique challenge.
What Does This Mean for the Future?
Understanding these challenges isn’t just about spotting the quirks in our current models—it’s about recognizing the progress that has been made and envisioning how future refinements might overcome these hurdles. Researchers are continually improving datasets, refining model architectures, and enhancing training techniques to help AIs better interpret spatial relationships and subtle details within human anatomy and symmetric designs. Each iteration brings us closer to AIs that are not only creative but also capable of producing art that meets our exacting visual standards.
Embracing the Imperfections
While it might be frustrating when an image generator doesn’t capture your vision perfectly, these quirks also underscore the unique blend of technical prowess and artistic interpretation inherent in these models. AI-generated art is a collaborative process—your prompts guide the creation, and the AI offers surprising interpretations based on its vast, data-driven understanding of the world. And who knows? Sometimes those unexpected twists might even spark a new creative idea.
Some examples
I wanted to create an image/cartoon of a magician pulling his own head out of a hat.

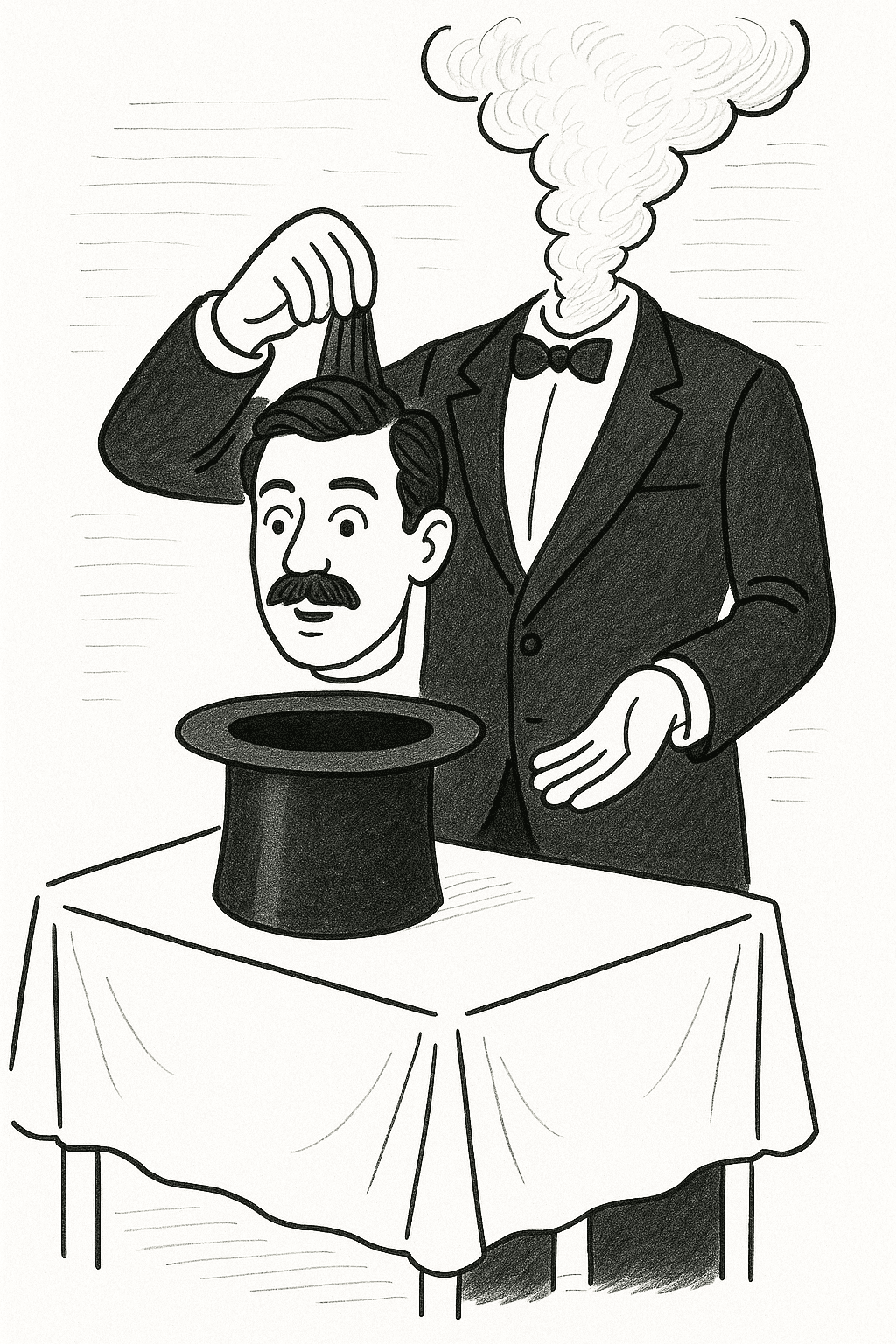
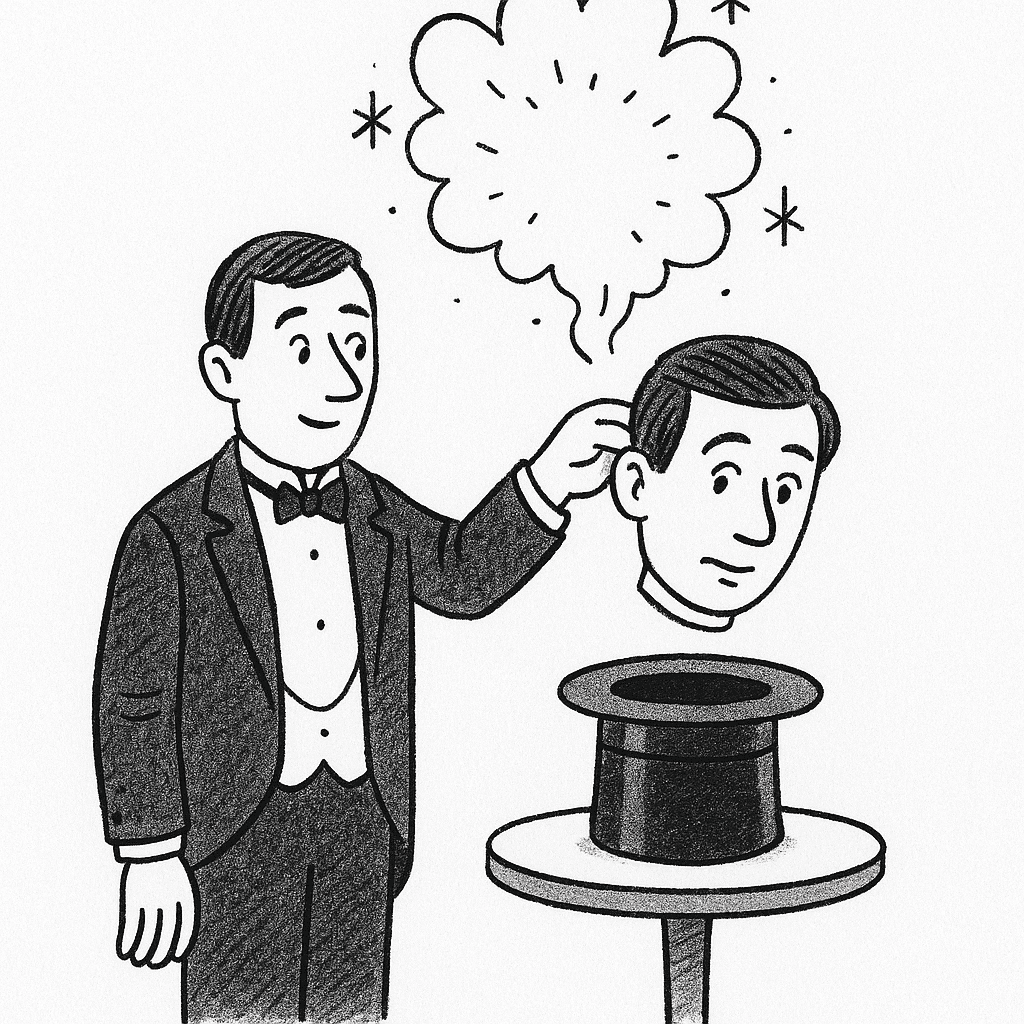